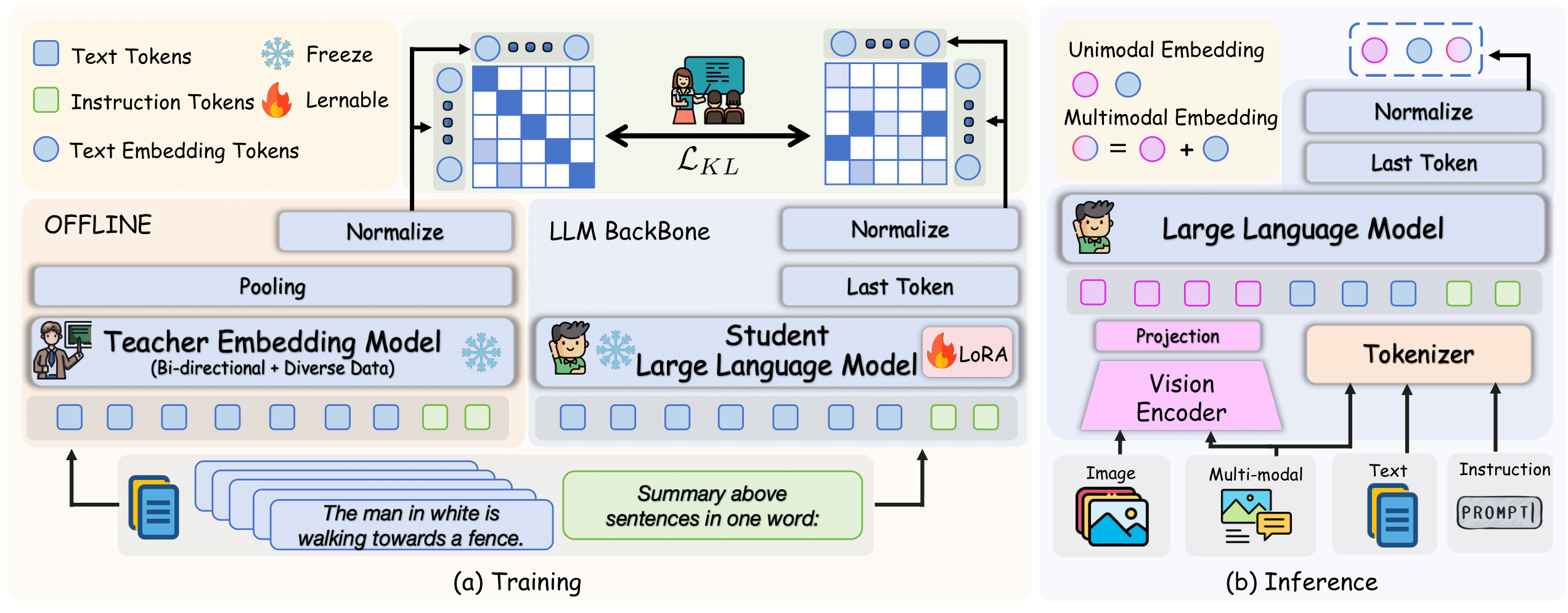
To enhance the MLLM's embedding capability, we propose textual knowledge distillation from NV-Embed V2 (a strong LLM-based embedding model). The training process involves decoupling the MLLM's LLM component and processing text with the prompt "Summarize the above sentences in one word.", followed by aligning the student (MLLM) and teacher (NV-Embed V2) embeddings via KL divergence on batch-wise similarity distributions. Notably, only the LLM component is fine-tuned during this process, while all other parameters remain frozen. During inference, the vision encoder is reintegrated for multimodal processing: unimodal inputs (text or image) use modality-specific prompts, whereas interleaved inputs generate the final representation by summing text and image embeddings.